The Faculty Outstanding MPhil Thesis Award recognizes and rewards an MPhil student each year, whose thesis has been identified by the Faculty’s Selection Panel. The prize will be presented at the Faculty Annual Awards Presentation Ceremony to be held in July 2022.
Mr. Li ‘s thesis titled “Irregular Deep Data Embedding and Learning” explores the applications of deep learning for data organized under the non-Euclidean structure, where two representative irregular data representations: graph, and point cloud are mainly studied.
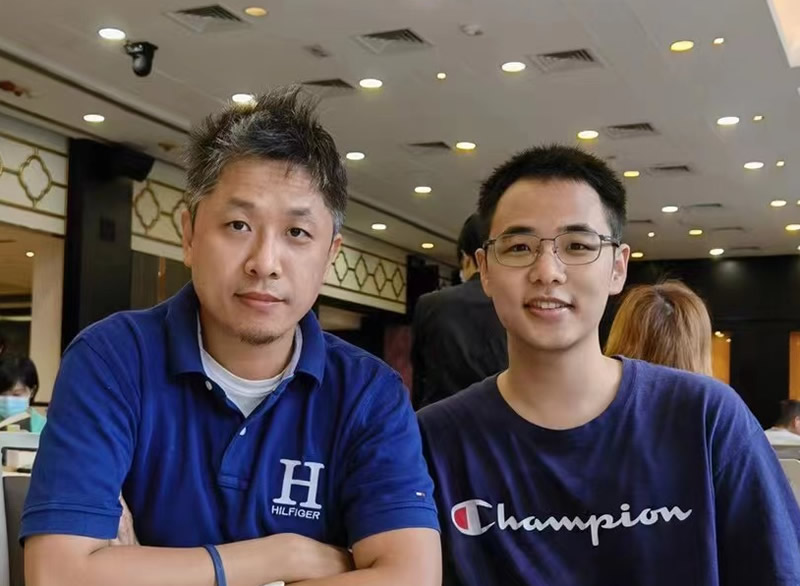
For the graph embedding, Mr. Li studied its applications in multiple patterning lithography decomposition (MPLD) problem and the graph coloring problem. He proposed a layout decomposition framework based on graph neural networks (GNNs) to obtain the graph embeddings of the layout. The graph embeddings were used for graph library construction, decomposer selection and graph matching. Besides the applications in the industrial workflow, he studied the power of GNNs for a pure graph coloring problem from the perspective of heterophily and locality.
For the point cloud embedding, he focused on its application to the routing tree construction problem. He modeled the pins of a net as a point cloud and formalized a set of special properties of such a point cloud. Considering these properties, he proposed a novel deep neural net architecture, TreeNet, to obtain the embedding of the point cloud. Based on the obtained cloud embedding, an adaptive workflow was designed for the routing tree construction.