Detecting COVID through Smart MachinesDou Qi develops AI algorithms that improve medical-image diagnosis for lung lesions
June 2021

The COVID-19 pandemic has put an enormous burden on healthcare systems. Thanks to Artificial Intelligence (AI) developed at CUHK, that load is a little easier to bear.
Prof. Dou Qi has developed a Deep Learning system that improves the detection of lesions in the lungs of COVID patients. Working with the team in her computer-science lab, she has designed a decentralized machine-learning system that can detect COVID-linked lung abnormalities in computed tomography or CT scans of the chest.
The findings connect three Hong Kong hospitals including Prince of Wales with counterparts in Germany and mainland China, for seven healthcare centres in all. The automated system uses ‘federated deep learning’ to coordinate lesion detection and share that data across multiple healthcare systems. The idea is to speed up the detection of lung lesions as a pandemic sweeps the world, and as knowledge of how to detect the disease improves in different locations.

Federated learning trains an algorithm to use machine learning across multiple decentralized servers, improving its intelligence without the hospitals needing to exchange patient data. Besides improving accuracy, it also protects patient privacy. The findings were published in late March in npj Digital Medicine, one of the open-access Nature Partner Journals.
Once the AI system has scanned for abnormalities in the lung, a doctor can check whether the AI prediction is correct or not. But having an AI pre-screening improves the accuracy, speed and efficiency with which a doctor can process many patients. Whereas a doctor may need anywhere from five to 15 minute to study a scan, the system can process one in seconds. The algorithm can also quantify the exact scope and severity of a lesion, in percentage terms, whereas doctors previously just gave their own personal interpretation that a case might be ‘mild’ or ‘serious’.

One of the challenges for using AI systems across a hospital network or internationally across borders is that the equipment used in each hospital may differ. That makes it difficult to compare information directly. Different hospitals use different machines with different settings and results.
‘Just like I have my phone and you have your phone, each phone takes a different image,’ Professor Dou explains. ‘The goal is to have one model that you can apply to many hospitals even though they’re using different equipment.’
The system delivered COVID lesion diagnosis within 95% accuracy. That’s better even than typical AI detection in cancer, which runs around 90% accurate.
The first applications of AI in health care have been to improve the detection of diseases such as cancer, and to improve the efficiency of the clinical staff. But Professor Dou also wants to push the boundaries of how AI is used. She believes many of the applications are only now emerging, or haven’t even been considered yet.
‘I’m very motivated to do these new things,’ Professor Dou says. ‘The first role of research is to find the problem, and define it.’
Professor Dou’s AI systems can also help improve imaging of the size and scope of a tumour. At the moment, an oncologist will very precisely draw the boundary of a tumour on a screen, to define its size and extent.
‘This kind of drawing is very time-consuming, taking as much as one to two hours for each patient,’ Professor Dou notes. ‘We provide the oncologist with the AI output, and then ask them to make their modifications on the AI output, to improve it.’
The system can cut the process of outlining the tumour to around 20 minutes. Over the course of a day, that allows for an improvement of at least 40% in terms of the number of cases that can be scanned.
Again, this has had application with COVID as well. The disease tends to produce multiple lung lesions, some large, many small. Describing the boundaries of each one would require minute detail from a clinician or doctor, and even then they may miss tiny lung imperfections. The AI system delivers that kind of detection in fine detail, allowing for the screening of a larger number of patients and with better results.
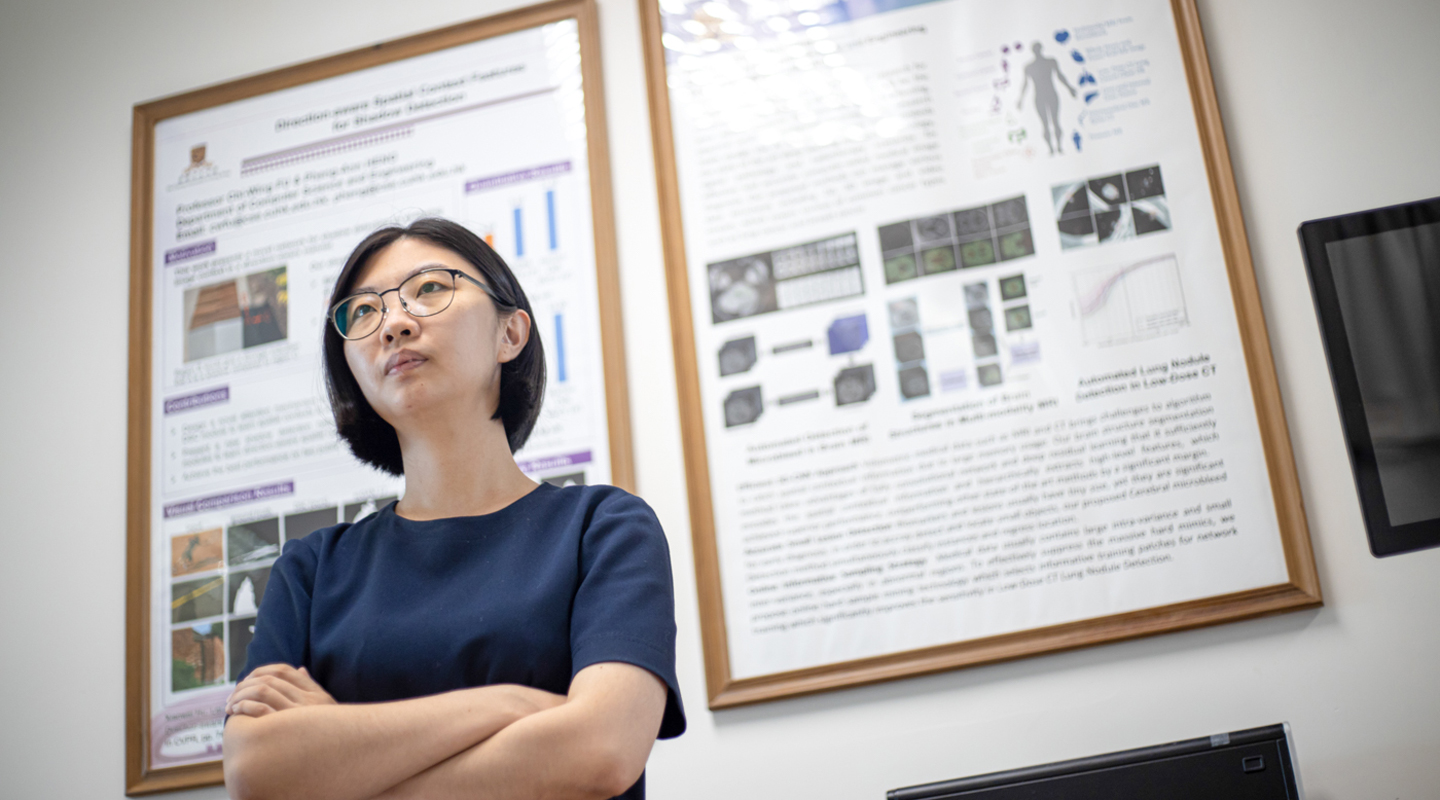
Professor Dou, who holds a post in CUHK’s Department of Computer Science and Engineering, is also working to develop algorithms that power the systems behind minimally invasive robotic surgery. That’s a very different type of surgery, where the doctor is in an entirely different location from the patient and the robot, which are attended by nurses. Everybody is watching multiple screens.
One of the current challenges for Professor Dou is to render the interior of a patient in 3D perspective in real time. For endoscopic surgery, the past approach has been to use an endoscope to provide a view inside the patient. But the surgeon is limited to seeing only what the endoscope is currently looking at. It’s one viewpoint at a time.
Professor Dou’s system uses the data delivered by the endoscope camera to form a 3D view of the patient and the surgery site, in real time. The endoscope is updating the image with new information, but the AI system retains previous perspectives to create a fuller picture of the patient.
This provides depth and perspective for the surgeon, who was otherwise relying on a 2D view. The AI system can also detect important features such as veins and nerves, so it can deliver an alert if the surgery is entering dangerous territory.
To date, patient safety depended entirely on the skill of the surgeon and the nursing staff, ‘eyeballing’ the surgery site. ‘If we put AI on top of that, it can serve as a backstop safeguard in the surgical environment,’ Dou says, helping improve the safety of the surgery in much the same way that image diagnostics are used to improve the accuracy of scans.
By Alex Frew McMillan
Photos by Eric Sin