Congratulations to Yonghao LONG (a PhD candidate supervised by Prof. Qi Dou) and co-authors (Jie Ying Wu, Bo Lu, Yueming Jin, Mathias Unberath, Yun-Hui Liu, Pheng Ann Heng and Qi Dou) won the IEEE ICRA 2021 Best Paper Award in Medical Robotics for the paper titled “Relational Graph Learning on Visual and Kinematics Embeddings for Accurate Gesture Recognition in Robotic Surgery “.
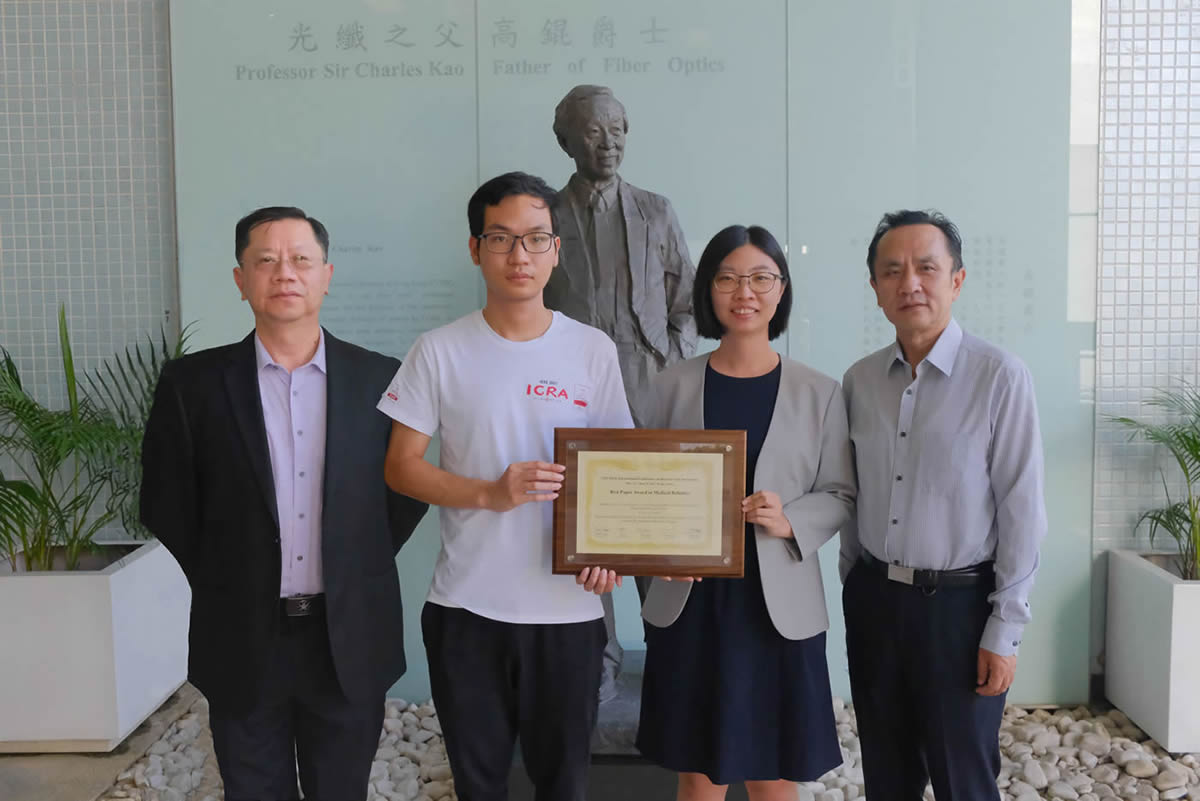
IEEE International Conference on Robotics and Automation (ICRA) is the largest and most important international academic conference in the field of robotics and automation. This award recognizes the best paper in Medical Robotics presented at ICRA 2021, where a total of 1690 papers have been selected for presentation, setting a new record in its history. The best paper award is judged based on aspects of technical merit, originality, relevance and potential impact on the medical robotics field, possible clinical efficacy, clarity of the written paper, and quality of the conference presentation.
Abstract:
Accurately recognizing robotic surgical gesture is important and fundamental to cognitive assistance and image-guided automation in robotic surgery. We newly develop relational graph learning techniques to integrate complementary information inherent in visual and kinematic multi-modal data recorded from the surgical robots for surgical gesture recognition. It is like jointly analysing the eyes and hands of the surgical robot to understand the complex procedure. This is the first attempt in the community to explore advanced AI techniques for correlating vision and kinematics, which opens a new door to significantly improve intelligent perception for medical robotics.
This project is jointly validated with Johns Hopkins University, Laboratory for Computational Sensing and Robotics, through international collaboration in Multi-scale Medical Robotics Center. And our developed AI system has been extensively validated on two sets of medical robotics platforms of da Vinci Research Kit (dVRK) systems established at CUHK and JHU. The AI model presents outstanding and stable performance on both platforms, demonstrating the promising generalizability and system independence, which is crucial for data-driven AI techniques to be widely incorporated in different medical robots in the field
